Association and causal mediation between marital status and depression in seven countries
Xiaobing Zhai (翟小兵)
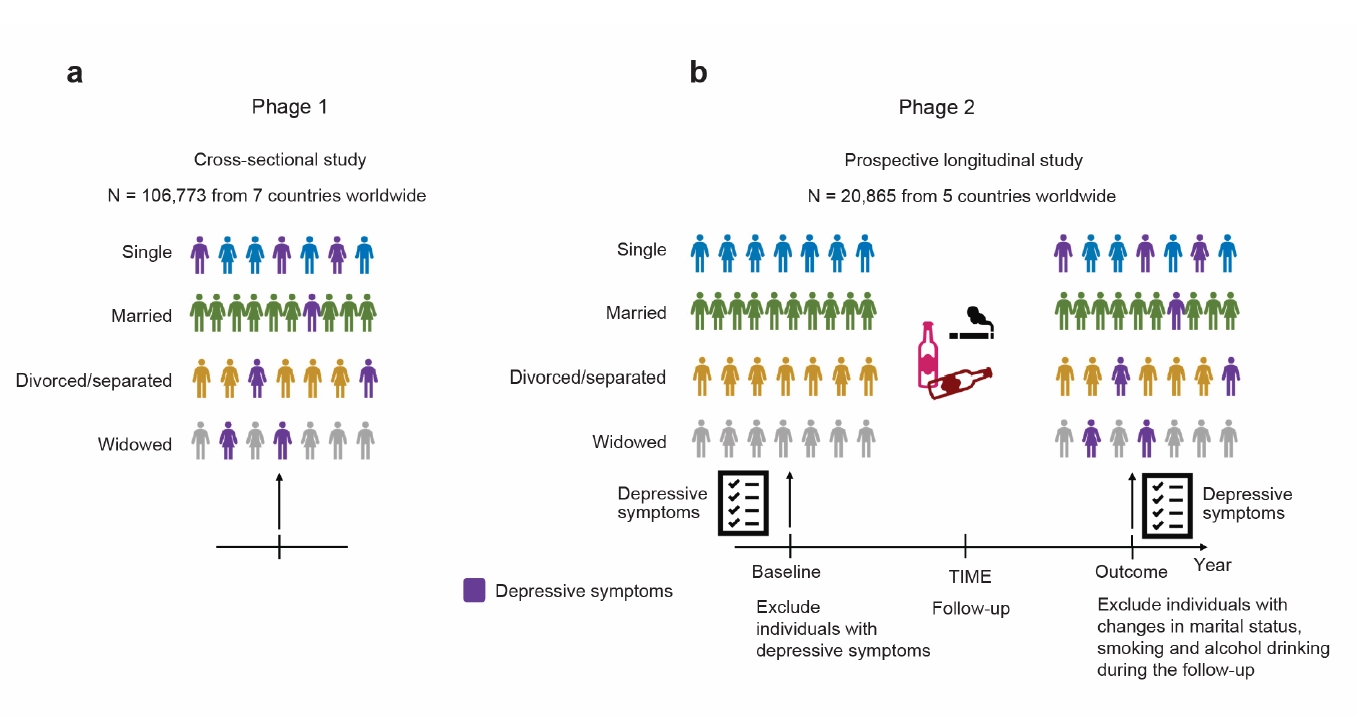
Depression represents a significant global public health challenge, and marital status has been recognized as a potential risk factor. However, previous investigations of this association have primarily focused on Western samples with substantial heterogeneity. Our study aimed to examine the association between marital status and depressive symptoms across countries with diverse cultural backgrounds using a large-scale, two-stage, cross-country analysis. We used nationally representative, de-identified individual-level data from seven countries, including the USA, the UK, Mexico, Ireland, Korea, China and Indonesia (106,556 cross-sectional and 20,865 longitudinal participants), representing approximately 541 million adults. The follow-up duration ranged from 4 to 18 years. Our analysis revealed that unmarried individuals had a higher risk of depressive symptoms than their married counterparts across all countries (pooled odds ratio, 1.86; 95% confidence interval (CI), 1.61–2.14). However, the magnitude of this risk was influenced by country, sex and education level, with greater risk in Western versus Eastern countries (β = 0.36; 95% CI, 0.16–0.56; P < 0.001), among males versus females (β = 0.25; 95% CI, 0.003–0.47; P = 0.047) and among those with higher versus lower educational attainment (β2 = 0.34; 95% CI, 0.11–0.56; P = 0.003). Furthermore, alcohol drinking causally mediated increased later depressive symptom risk among widowed, divorced/separated and single Chinese, Korean and Mexican participants (all P < 0.001). Similarly, smoking was as identified as a causal mediator among single individuals in China and Mexico, and the results remained unchanged in the bootstrap resampling validation and the sensitivity analyses. Our cross-country analysis suggests that unmarried individuals may be at greater risk of depression, and any efforts to mitigate this risk should consider the roles of cultural context, sex, educational attainment and substance use.